Are you a data quality champion or a data quality chump?
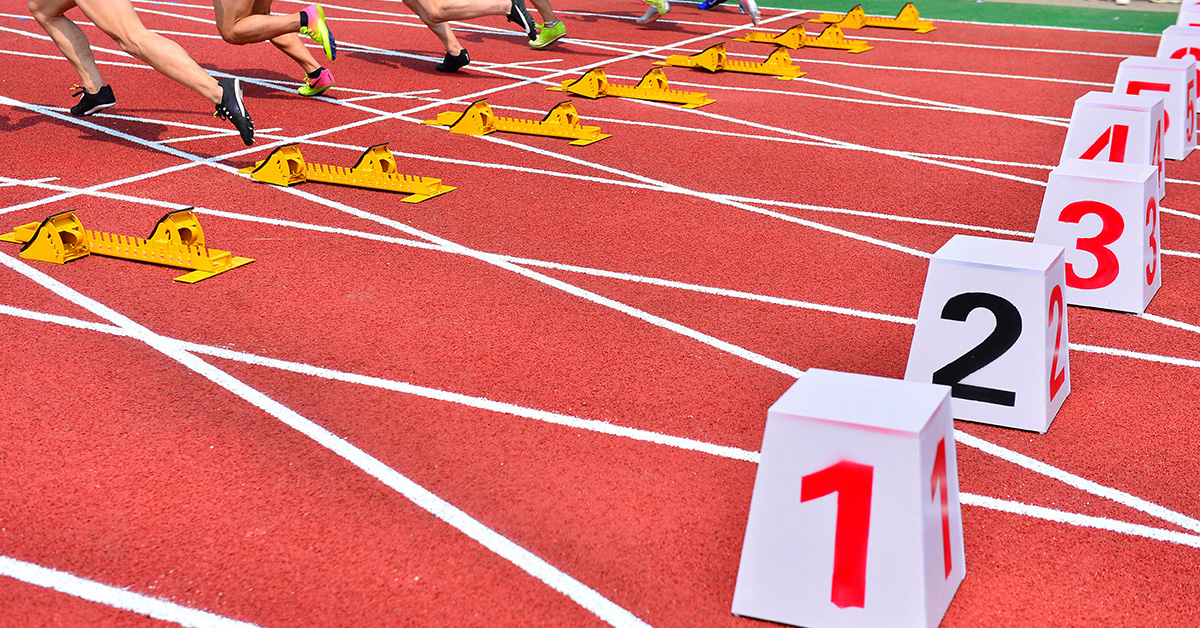
Key takeaways
- Data quality benchmarking helps to identify gaps in an organization's data quality processes and target their improvement efforts more effectively.
- Exemplary benefits of data quality measurement and benchmarking of business partners are transparency, efficiency, data excellence, and self-promotion.
- Benchmarking data quality with other companies can provide valuable insights and inspiration for organizations looking to improve their data quality and establish themselves as leaders in their industry
The daily pain with data quality
Dear Data Management Department,
I am writing to you in complete outrage and frustration regarding the appalling state of data quality in our ERP. It has come to my attention that a significant number of EU VAT identifiers are missing, outdated, or just plain wrong, which poses a serious risk of violating rules for tax exemption.
I expect that our data management should have been more vigilant in ensuring that the data in the system is accurate and up-to-date. However, it appears that this is not the case, and data governance has failed miserably.
The consequences of this kind of negligence can be catastrophic, both for our business and our partners. We are at risk of tax claims, penalties and legal action, not to mention the damage to our reputation and credibility.
I demand that you take immediate action to rectify this situation. The data stewards must be held accountable for their lack of attention to detail, and measures must be put in place to prevent this from happening again in the future.
In summary, I expect swift and effective action to be taken, and the consequences for not doing so will be severe.
Sincerely,
XXX
Uff... ever experienced such kind of mailing? Validation and proper management of business partner data (i.e. customers and suppliers) is crucial!
- Do you know how many really active business partners you have maintained in your systems (CRM, ERP, ...)?
- Do you know the level of quality of your business partner data? (NOT gut feeling or estimations, but hard facts!)
- Do you have any idea how your performance in data management compares to other organizations or even key players in your industry?
- Have you ever thought about continuously measuring data quality, setting up a data quality cockpit and benchmarking your business partner data quality with other organizations?
If one of these questions triggers you, then the following will hopefully raise your attention!
Why should you measure and benchmark your business partner data quality!
Data quality measurement is a valuable tool for organizations looking to improve the accuracy, completeness, consistency, and timeliness of their business partner data. Moreover, it creates transparency and enables to monitor the compliance with regulatory requirements. It should be part of any data strategy.
Data quality benchmarking of business partner data quality allows organizations to compare the performance of their business partner data management with other companies in their industry.
As Malcolm Hawker states in his Gartner blog post from 2020:
If you’re only comparing your enterprise data to itself, how do you know if it’s any good?
(Read Malcolm Hawker's blog post here)
So, data quality benchmarking helps to identify gaps in an organization's data quality processes and target their improvement efforts more effectively. Moreover, by data quality benchmarking against industry peers, organizations can identify best practices, build trust and credibility with their stakeholders, and identify potential risks and opportunities.
Some overview on the benefits of data quality measurement and benchmarking I give below:
Transparency and Efficiency
- Benchmarking business partner data quality can also help organizations to identify potential risks and opportunities related to data quality. For example, if benchmarking results show that data quality is declining for certain types of business partners, organizations can take proactive measures to address the issue before it becomes a problem.
- With data quality benchmarking, you can eliminate investment decisions based on gut feeling and instead rely on actual data. You can measure the performance of data governance and data quality activities, and receive an early warning when data quality drops for certain countries or data objects.
- Measuring business partner data quality helps organizations to monitor their compliance with regulatory requirements, such as Know Your Customer (KYC) and Anti-Money Laundering (AML) regulations. By benchmarking against industry peers, organizations can ensure that they are meeting the same standards as their competitors and avoid potential penalties or reputational damage.
Data Excellence
- By publicly sharing benchmarking results, organizations can demonstrate their commitment to data quality and build trust and credibility with their customers, partners, and other stakeholders. This can be particularly important in industries where business partner data quality is critical, such as finance, healthcare, and manufacturing.
- By benchmarking against industry peers, organizations can identify best practices for managing business partner data and learn from others' successes and failures. This can help them to improve the quality of their business partner data and reduce the risk of errors or omissions.
- Some companies use data quality benchmarking to set targets for their employees' MbOs (management by objectives). This approach encourages employees to strive for excellence. Additional gamification approaches may improve the overall performance of the organization. One of our customers set the goal of being the number one in business partner data quality for European customers and vendors.
Self-promotion
- You can communicate your organization's excellent data management performance to stakeholders. By using data quality benchmarking, you can showcase your organization's achievements and demonstrate the need for investment in data governance and necessary tools.
- Benchmarking can also be used to identify areas where organizations are outperforming their peers in terms of business partner data quality. This can help them to leverage their strengths and identify opportunities for further improvement.
Additional use cases for rule based data quality measuring
Besides this there are even more specific use cases for setting up a rule based data quality measurement
Data Quality Assurance
Avoid data quality issues when creating and maintaining data by early detection of errors. Sometimes also referred to as First Time Right. The later you reactively fix data quality issues, the higher your effort, so better strive for First Time Right.
Santosh Kudva states in the below linked article:
The best option with the highest business impact with the least cost and no risk is to get it ‘first time right’. Get the data right when the data is created or updated.
(Read Santosh Kudva's article here)
Data Maintenance Automation
A case for the more mature organizations that already have set up a certain level of data governance. They can become more efficient by for example skipping unnecessary approval workflows. Data quality rules ensure here the required level of trust in the data.
A great application of this approach I implemented with Bayer AG. They won the CDQ Good Practices Award in 2020. Watch the following video:
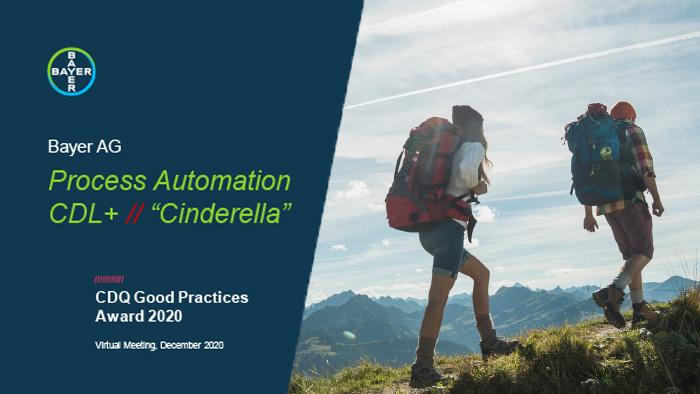
VAT Compliance in the European Union
Companies need to include all EU VAT identification numbers of their customers in their ERP systems! Validating VAT identification numbers at least periodically or before each shipment and properly document these controls! Even better is to continuous monitor the VAT registrations of all customers in the system.
Rule based real-time validation of EU VAT Numbers of all member states allows for accuracy and compliance. If you want to read more, then I recommend reading my article Stay clear of tax hurdles: Why validating European VAT Numbers is key for your business
How to measure data quality?
Data quality is described using data quality dimensions, as it is a complex construct that can vary depending on the context in which it is used. These data quality dimensions are used to reflect the quality of data based on its fitness for a specific use, as assessed by a particular data consumer. Just like a low budget drill may be sufficient for hanging an IKEA shelf, but not for a professional renovation project, data can be of sufficient quality for one task but of too low quality for another.
To be clear here, my intention is definitely not to write yet another article about data quality dimensions and their definitions. It's worth noting that data quality dimensions have been around for over two decades now, and there are many great resources available on the topic. One very frequently cited research article on the topic by Richard Wang and Diane M. Strong dates back to 1996, but really aged well: Beyond Accuracy: What Data Quality Means to Data Consumers
What role do data quality rules play?
Data quality rules are essential for ensuring that data is accurate, consistent, and reliable. They are used to define standards and constraints that data must adhere to, and help organizations maintain data integrity.
But how do they help me when I need to measure data quality? Well, measuring data quality is not as simple as measuring an object with a ruler. Unlike having a ruler, measuring data quality may require a significant investment of effort and resources upfront. It's like first inventing the ruler before you can start measuring. Organizations need to define and implement data quality rules that outline the standards and constraints data must adhere to. This requires deep analysis of the business requirements on the data.
A pure measurement of the data quality dimension completeness will usually be totally ineffective. Imagine you always maintain streets with house number in one data field. Is it really a relevant data quality issue to measure now the completeness in terms of having the house number field filled? Might be nice to have it, but is it really any requirement of a consuming business process?
We at CDQ developed a standard ruleset for business partner data quality with more than 2'000 data quality rules. This one we use for measuring and benchmarking the data quality of our customers. Check out the following article if you are interested in more details: How data quality rules help you to make informed business decisions
CDQ Data Quality Cockpit
Measure and benchmark your data quality with industry peers!
Setting up the CDQ Data Quality Monitor for Business Partners is simple:
- Sync your business partners with the CDQ Cloud (API or App)
- Configure and activate the CDQ Data Quality Monitor (API or App)
- Analyze your data quality and benchmarking performance in a dashboard, download data quality reports or integrate via our API
Data quality overview
Below you can see the overview page, giving you a glance on your overall data quality and its change over time.
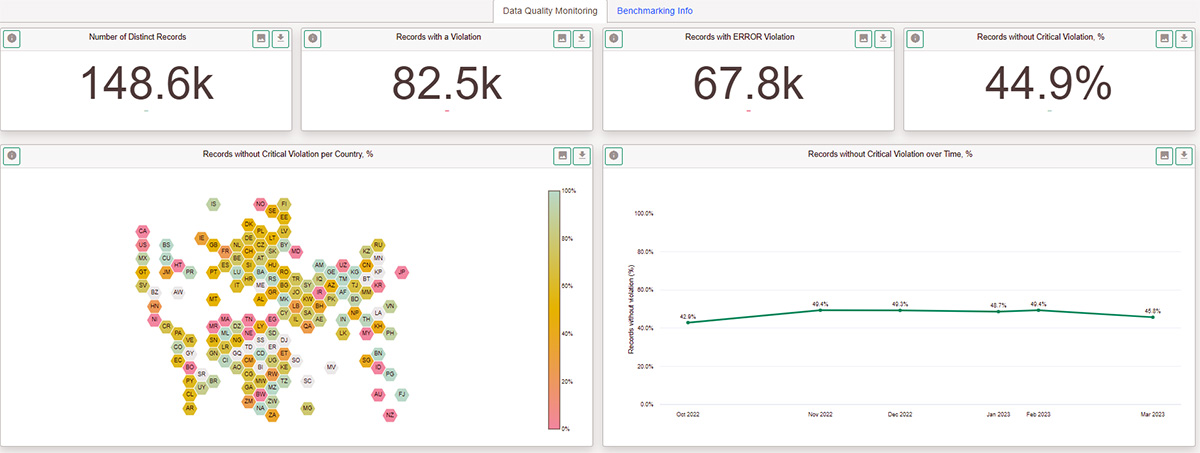
Benchmarking overview
The benchmarking view compares your data quality with other companies:
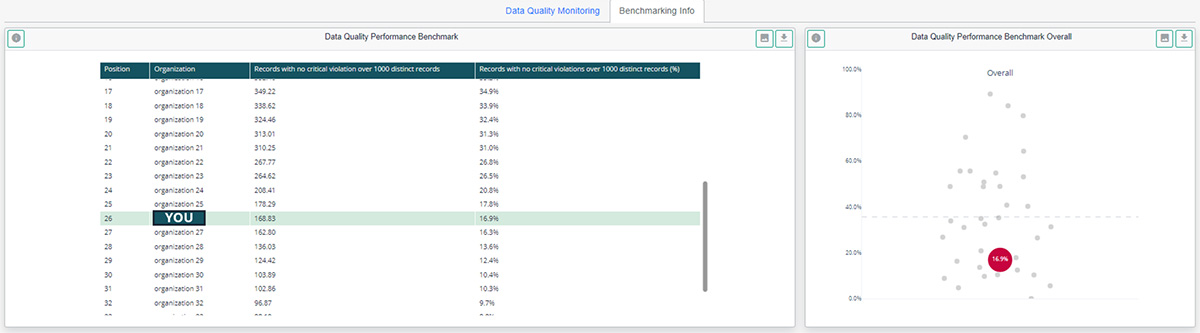
An additional world map shows the average data quality of all companies for each country:
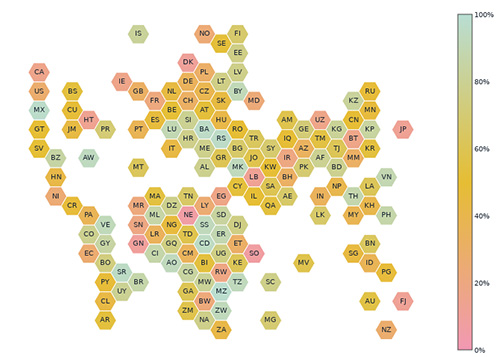
Reviewing data quality issues
Filter and review business partners with data quality issues:
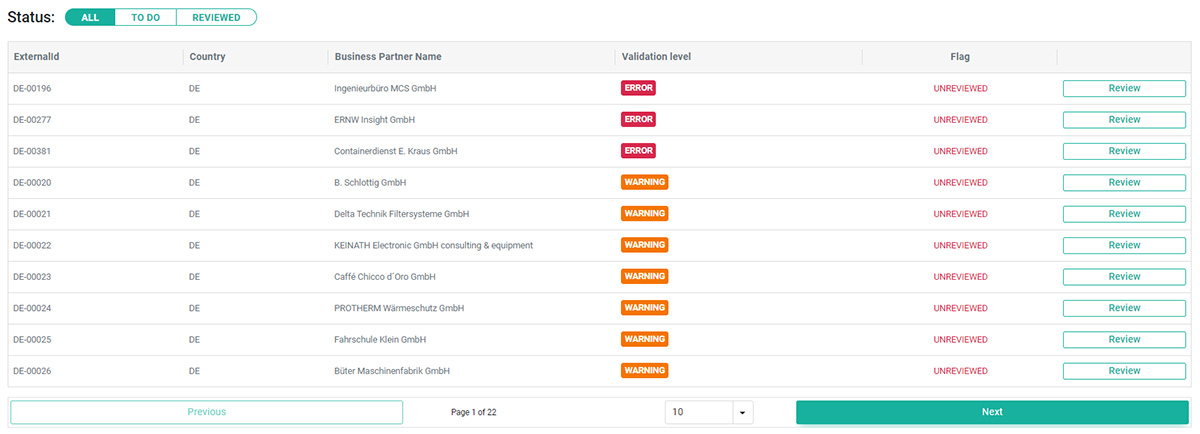
Exemplary view on violated data quality rules:
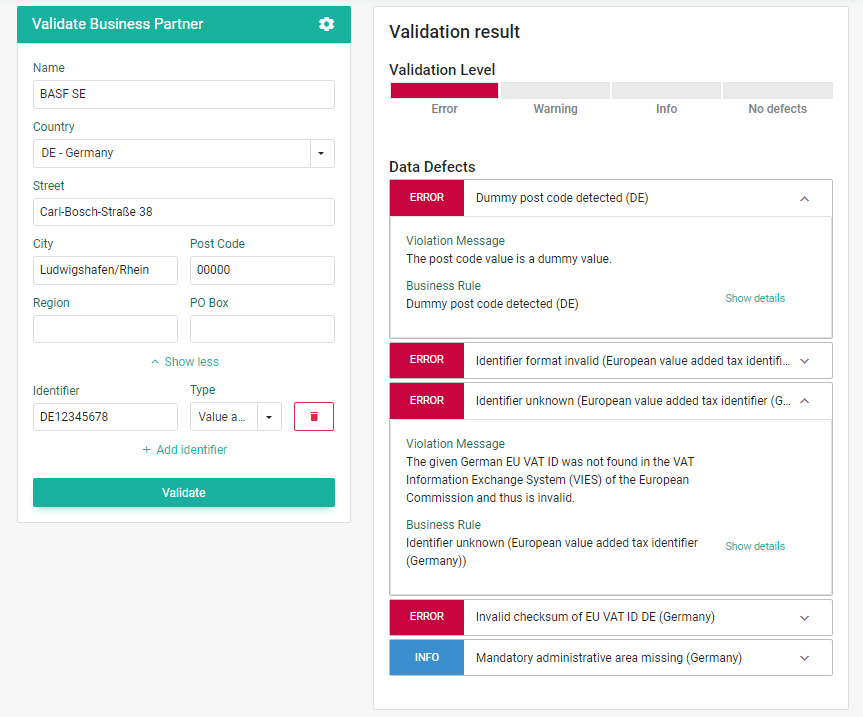
Ok, if I know then that I'm a chump... how can I improve?
CDQ's Cloud Suite for Business Partners enables you not only to measure and benchmark, but helps you to correct the data as well! Moreover, it allows for continuously monitoring for data updates in external data sources and the data sharing community!
Interested?

Get our e-mail!
Related blogs
Even more data quality rules available out-of-the-box
At CDQ, we take data quality seriously. And at the core of our data quality journey lies the active involvement of our Data Sharing Community. We believe that…
The hidden costs of duplicate business partner records
Duplicate business partner records can have a significant impact on organizations, leading to hidden costs that can hinder operational efficiency and decision…