Data Quality
Is your master data fit for purpose?
… the data reality proves otherwise
critical violations
outdate within 1 year*
unintended duplicates
The problem with data quality
In the digital economy, the role of data is changing. The quality of your master data influences every facet of business operations and decision-making. But with data evolving rapidly, maintaining reliable information becomes a challenge.
Good quality business data in real life
In the dynamic landscape of modern business, your company's success hinges on accurate, reliable, and actionable data. We understand the struggles you face every day when dealing with data maintenance and creation challenges due to poor data quality. That's why we're here to guide you towards a transformational solution that will elevate your data game and empower your business for the digital age. Tackle your Corporate Data Quality with CDQ!
Value of Automation in Master Data Management
Get our free eBook and explore how automation can unlock significant cost saving for your organization. Turn master data challenges into financial assets through intelligent automation and revolutionise the way you manage business partner data!
See real-life examples of saving big with CDQ cloud solutions and turn your burden into a financial asset, just like others did already - click on the button below to download your copy, so you can unlock instant value.
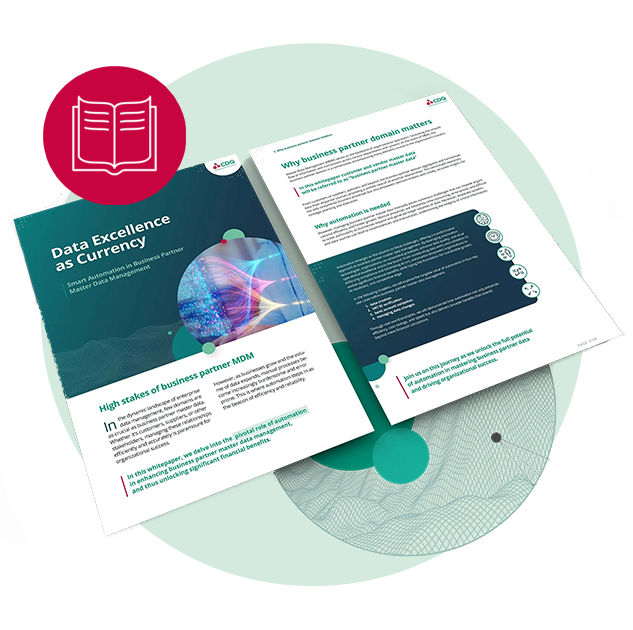
Business impacts of your data quality
The power of data is undeniable—it fuels core processes, empowers decision-makers, enhances customer experiences, and drives overall business success. However, the true potential of data can only be unlocked when it meets the high standards of accuracy, completeness, and reliability.
In the absence of automated data creation and maintenance, the occurrence of data errors becomes more than just an inconvenience – and especially in the field of business partner data. The repercussions of bad data ripple across the entirety of business functions, and the consequences are far from trivial, as the very foundation of your business processes and relationships begins to crumble.
The Anatomy of Data Quality
Data quality is a measure for the suitability of data for specific requirements in your business processes.
A low level of data quality will reduce the value of the data assets in the company because its usability is minimal. Data quality characterizes the degree of how given data objects satisfy the needs of consuming business processes. In a broader sense, it refers to both the quality of data content as well as the performance of the underlying data management processes.
Additionally, data quality measurement gives you transparency and enables to monitor the compliance with regulatory requirements.
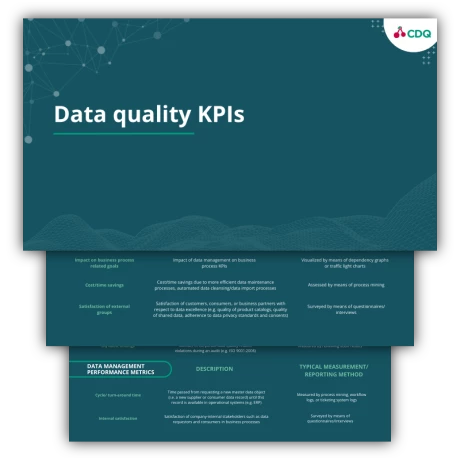
Data Quality Dimensions
Your data fitness for use extends beyond its mere content – it's also about the processes that govern it. Does your data quality touch all the critical dimensions?
- Does it reflect the full business landscape or leaves vital details in shadows?
- Is it consistent as it flows through diverse touchpoints in your operations?
- Is the accuracy and alignment of your data with predefined standards a constant guarantee?
- Is your data free from duplicates?
- Are all data users happy with the timeliness and accessibility of the data?
At CDQ we believe that high data quality is synonymous with data excellence and we’re on a mission to make your data quality thrive. Whether enterprise-migration to SAP S/4HANA or legacy systems causing you headaches, we’ve got you covered.
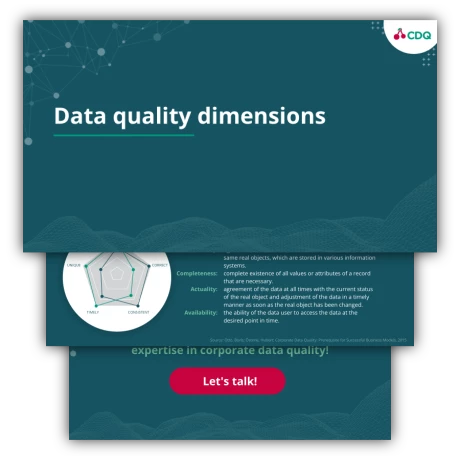
Apply Data Quality as a Service
Imagine a world where data maintenance is no longer a cumbersome task, but a seamless and automated process ensuring good data in your downstream processes. That's the world we're creating with the innovative CDQ Suite – your gateway to Data Quality as a Service (DQaaS).
It’s a quick, easy-to-use, and fully automated process to validate, enrich, and cleanse your data. Thanks to deep integration into customers’ backend systems, the CDQ suite improves data quality right at the source, so you can avoid manual mistakes and lower maintenance efforts.
Access 70 external data sources and more than 2500 ready-to-use data quality rules powered by data sharing to elevate your master data quality.
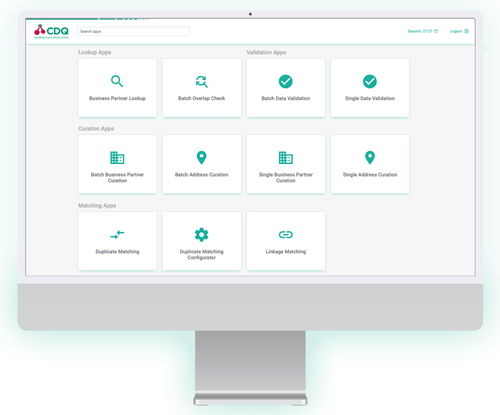
Apply quality to your master data with us: in-business and in-class
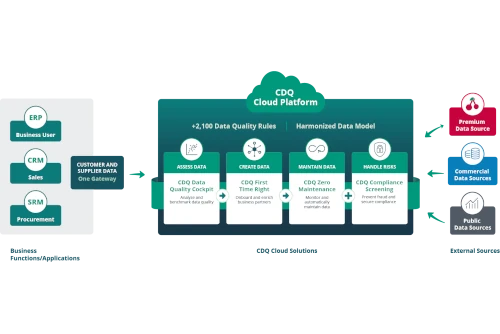
CDQ Cloud Platform is a single gateway to 70 external data sources and more than 2500 ready-to-use data quality rules powered by data sharing and provides easy-to-integrate capabilities for your data journey from the start.
“We could deliver faster and better! We are happy to embark on the next part of our data quality journey with them!”
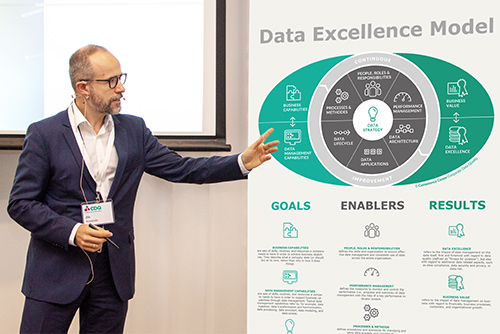
The CDQ Academy is a practical three-module master data management training. Since 2013, more than 700 participants have benefited from the data quality management expertise of CDQ and the University of Lausanne.
“You will get a better understanding on the backside of what customer expectations to the data quality are.”