Data Mesh and the case of Data Sharing
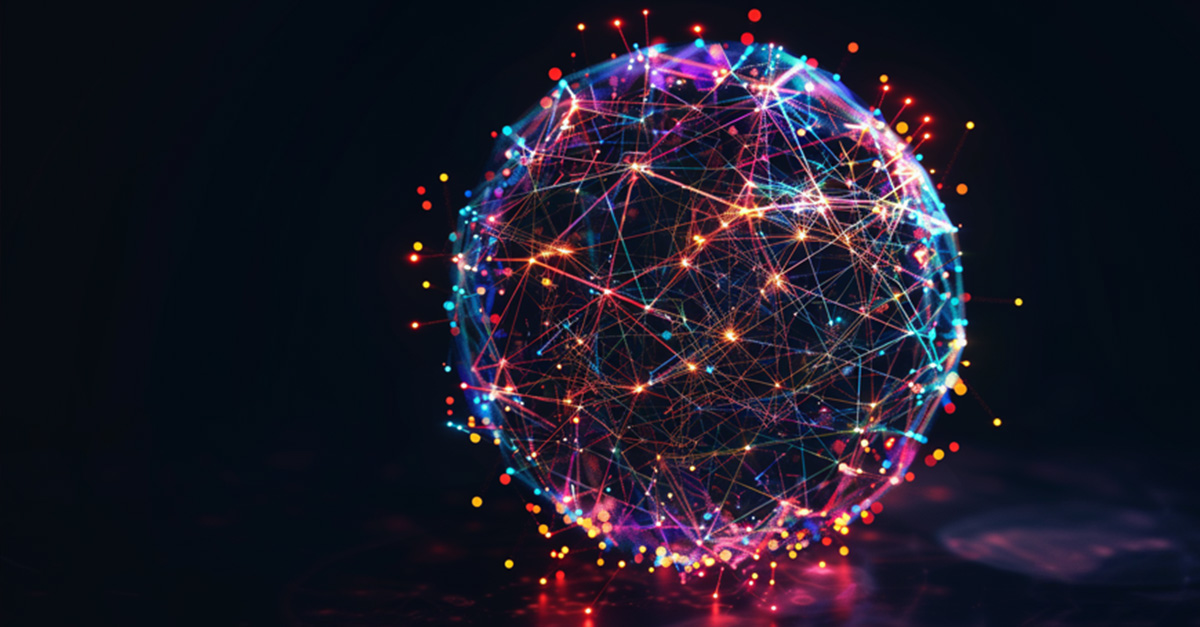
Fueled by the increasing demand for distributed, scalable, and agile data platforms that can enable data democratization and innovation across organizations, data mesh is a widely discussed topic.
“Data mesh” hereby refers to a distributed architecture for managing and analyzing large-scale data across multiple domains and teams. It aims to overcome the limitations of centralized data lakes and warehouses by enabling each domain to create, own, and share its own data assets in a self-service manner, while ensuring interoperability and governance through common standards and protocols.
While it is a promising concept, some challenges data mesh poses should be considered:
1. Data governance and quality:
The data mesh concept requires a balance between autonomy and coordination, and between flexibility and standardization. It also poses new challenges for ensuring the quality, reliability, availability, and security of the data assets across distributed systems, and for resolving potential conflicts and inconsistencies among different data sources and schemas.
2. Data culture and literacy:
Data mesh requires a shift in data culture and mindset of the organization, from a centralized and siloed approach to a decentralized and collaborative one. It also requires a higher level of data literacy and awareness among the data producers and consumers, who need to understand the value, context, and usage of data they create and access, and have to adhere to the data mesh principles.
3. Data infrastructure and technology:
The data mesh idea requires a robust and scalable data infrastructure and technology stack that can support the distributed, heterogeneous, and dynamic nature of the data architecture, and enable data sharing and interoperability across domains. It also requires continuous monitoring and optimization of data performance and costs, and rapid adaptation to changing data needs and demands.
Data Mesh and Data Sharing – it’s a match
At CDQ, we strongly believe in “Data Sharing is the best way to better data”. This philosophy aligns seamlessly with the data mesh concept, emphasizing decentralized data ownership, domain-specific control, and a collaborative approach to data management. As a leading enabler for data ecosystems, CDQ leverages the data mesh framework to enhance data sharing capabilities within these networks, thereby addressing and bridging semantic gaps between various external data sources and corporate standards.
Here's how the data mesh approach strengthens our mission.
- Decentralization and Domain Ownership: Data mesh advocates for decentralizing data ownership to specific domains within organizations. CDQ empowers these domains by providing tools and platforms that facilitate the sharing and integration of business partner data. This approach ensures that data remains close to its source, maintaining its context and quality, which is vital for effective data ecosystems.
- Data as a Product: By adopting the data mesh principle of treating data as a product, CDQ enhances the value of shared data. We help organizations to structure, document, and share their data in a way that is usable and valuable to others within the ecosystem. This product-oriented approach improves the quality, usability, and accessibility of data across different stakeholders.
- Self-Serve Data Infrastructure: Data mesh promotes a self-serve data infrastructure, empowering users to access and utilize data independently. CDQ supports this by providing a platform that enables easy access and interaction with shared data, fostering a culture of self-service and autonomy among business partners and clients.
- Interoperability and Integration: In line with data mesh principles, CDQ focuses on ensuring interoperability between different data sources and systems. Our solutions aim to bridge semantic gaps, ensuring that data shared across the ecosystem is understandable and actionable for all participants, despite varying corporate standards and formats.
- Governance and Compliance: Data mesh does not neglect governance; instead, it integrates governance into the fabric of the data ecosystem. CDQ upholds this principle by offering governance frameworks and tools that align with decentralized data ownership, ensuring that data sharing remains compliant with industry regulations and standards while respecting domain-specific requirements.
- Collaboration and Community: The data mesh model fosters a collaborative environment by encouraging domains to share data and insights. CDQ champions this community-driven approach, facilitating collaboration across corporate boundaries, enhancing collective intelligence, and driving innovation within the data ecosystem.
- Enhancing Data Literacy: By promoting data sharing and collaboration, CDQ also supports the improvement of data literacy across organizations. The data mesh framework encourages a deeper understanding and engagement with data, which CDQ facilitates through educational resources, support, and community building.
- Improved Data Quality and Reliability: By adopting a data mesh approach, companies can improve the quality and reliability of business partner data. As each domain is responsible for its own data, there is a natural incentive to maintain high data standards. This results in cleaner, more accurate data, which is crucial for businesses relying on this information for decision-making and operations. Providing high-quality, reliable data can set a service apart from competitors.
- Faster Data Access and Reduced Time-to-Insight: Data mesh enables faster access to data by decentralizing data ownership and management. This reduces bottlenecks and delays in data access, allowing businesses to gain insights more quickly. For data sharing services, offering rapid access to high-quality business partner data can be a significant competitive advantage.
If a certain company, one of our customers, already has a data mesh established, they can significantly extend and enhance this mesh through CDQ services in the following specific ways:
- Integration of Trusted External Data Sources: CDQ services can extend a company’s existing data mesh by adding trusted external data sources. This means integrating data from various external sources such as trade registers, financial institutions, and ESG rating agencies directly into their Data Mesh. This integration ensures that the company's Data Mesh not only contains their internal data but also rich, validated external data, providing a more comprehensive view of business partners and market contexts.
- Reliable Inbound Data Stream: Through CDQ services, companies can establish a reliable inbound stream of continuous updates for their own business partner data. This ensures that the data within their data mesh is not static but continuously refreshed and updated, keeping it relevant and accurate. This dynamic data flow is crucial for maintaining the integrity and value of the Data Mesh over time.
- Cross-Corporate Collaboration Enhancement: By leveraging CDQ’s cross-corporate data collaboration platforms, a company can further extend its data mesh beyond organizational boundaries. This facilitates data sharing and enrichment across different companies, enhancing the collective data quality and usability. This extended collaboration can lead to a larger, interconnected data mesh that spans across our entire customer base and beyond.
- Standardization and Semantic Harmonization: CDQ services help standardize and reconcile data semantics across different data sources and corporate standards. This is essential for a functioning data mesh, as it ensures interoperability and meaningful data exchange within and between different meshes. By using CDQ services, companies can ensure that their extended data mesh operates seamlessly, with standardized data formats and definitions across the ecosystem.
From an outside perspective, the individual data meshes of our customers, when interconnected through CDQ’s services, form a larger, global data mesh. This global mesh combines internal corporate data with external trusted sources, enriched by the collective intelligence and contributions of all participating organizations. This expansive mesh not only enhances the data capabilities of individual companies but also contributes to a larger ecosystem of shared, high-quality data.
Last, but not least we would like to share a few tips on how businesses should adapt to leverage the impact of the data mesh
- Assess your current data maturity and readiness for adopting the data mesh, and identify their key data domains, assets, and use cases that would benefit from the data mesh approach.
- Establish a clear vision, strategy, and roadmap for implementing the data mesh, and align their data goals and objectives with their business outcomes and value proposition.
- Foster a data-driven culture and mindset across your organization empowering and incentivizing data teams and stakeholders to collaborate and innovate with the data mesh.
- Invest in building and maintaining a data mesh infrastructure and technology stack that can support their data mesh needs and requirements, and leverage the existing or emerging data mesh frameworks, tools, and solutions in the market.
- Implement and enforce a data governance and quality framework that can ensure the trustworthiness, security, and compliance of their data assets across the data mesh, and monitor and measure their data performance and impact.
Summing up, data mesh can resolve limitations of traditional centralized data architectures, such as data lakes or warehouses which often suffer from silos, bottlenecks, and low-quality problems.
Whether data mesh or a classical architecture is your favorite approach to achieve accessibility, usability and quality of data will depend on individual factors such as size and complexity and also compliance questions in your specific network.
The goal though should always remain the same: empowering data owners and consumers to collaborate and share data as self-contained and interoperable data products.
Get our e-mail!
Related blogs
Eine Deutschland AG der Daten: the time is now
In their thought-provoking guest article in the Frankfurter Allgemeine Zeitung, our CDQ colleagues Prof. Dr.-Ing. Boris Otto and Dr. Sebastian Muschter argue…
Unlocking the power of Data Sharing: the CDQ approach
For nearly a decade, CDQ has been leading the way, pioneering a secure and collaborative approach to business partner data sharing. With our innovative Data…
From e-bikes to e-mail guards: CDQ Data Sharing Community Workshop highlights
Another unforgettable CDQ Data Sharing Community Workshop has wrapped up, and what an incredible two days it’s been! Packed with insightful sessions, engaging…