Data quality rules: the fuel your master data management runs on
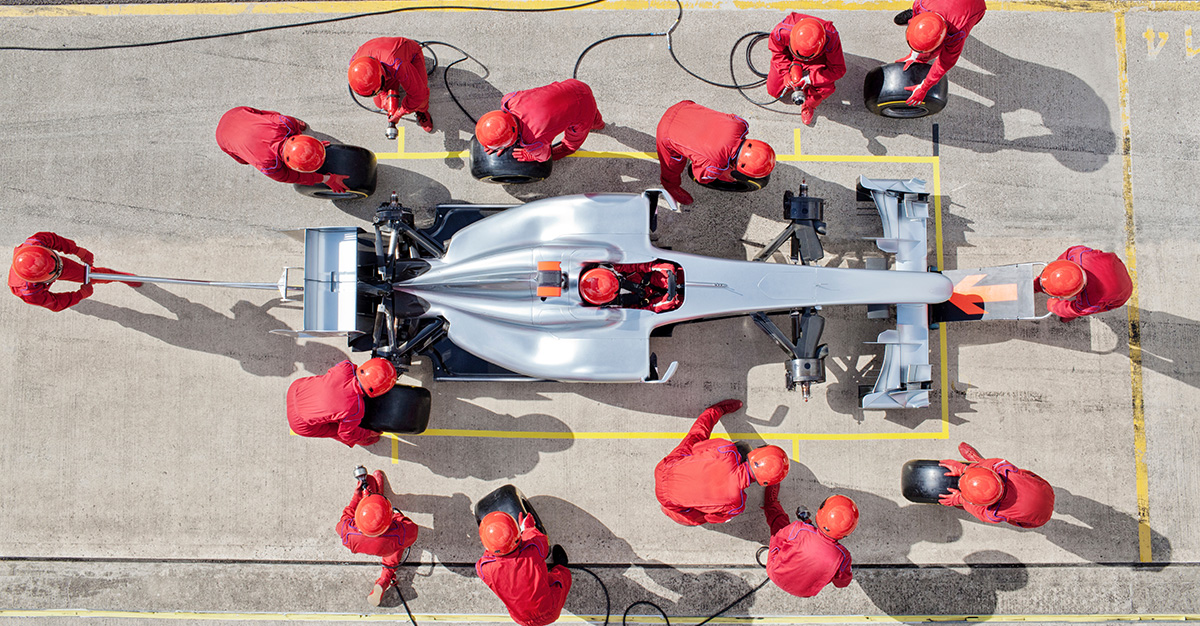
The world of data quality rules offers a fascinating journey. Mine started when I realized how bad the business partner data quality is at organizations. During my PhD at HSG University of St.Gallen, I was leading a large government-funded project. We were identifying the potential and designing a solution for cross-corporate business partner data management and that was a pretty intense deep-dive into the topic of data quality rules.
I found that there are easy mechanisms to understand, analyze, improve and avoid many data quality issues with clearly defined data quality rules. As a researcher, I understood the importance of rules and procedures for meeting at least a minimum level of quality.
But it wasn't until I started working with data stewards sitting in shared service centers across the world that I really saw the need for shared rules to enable shared ownership on business partner data.
Can you guess, how a data steward sitting in a shared service center in Hyderabad, India knows how a German address is composed and can be validated? Or vice versa, how a German data steward knows about the specialties of the Japanese address system and how to maintain it correctly in SAP?
Well, neither could I... And to make things worse: data stewards were of course confused too.
But one thing was clear: rules and procedures are required for meeting at least a minimum of data quality.
What exactly is a data quality rule?
To understand the importance of data quality rules, it's essential to know what a data quality rule is. A data quality rule put simply, is a mechanism that allows analyzing whether a data record a data record is fit for purpose and meets the business requirements to be used in business processes. Many people mix up the terms "business rule" and "data quality rule," but they are not quite the same. A business rule formulates the business requirement in business language, while the data quality rule formulates and implements the business requirement focusing on the data.
So, how does the process of formulating a data quality rule go? It involves:
- understanding and analyzing the business requirement
- identifying and collecting required reference data
- implementation (in terms of transferring business requirements into a data quality rule)
- and testing
Sufficient number of rules depends on the business purpose of the data, and the definition of fit for use differs.
But a bare minimum is that any data attribute should be checked by at least one data quality rule. There is some correct value that could be entered in a particular field, or not, and so it should be somehow checkable if machines are meant to be more clever than humans.
Top rules
One of the most often used data quality rules is the validation of VAT identifiers, and it's a critical rule that helps our clients ensure high-quality data already when data enters the system. This is just one example of many rules that we have developed over the years, amounting to 2156 in total as of today.
In the past 12 months, we developed over 200 new rules. And our Data Sharing Community plays a critical role in this process: the community formulates business requirements or required improvements, and our team translates these requirements into data quality rules.
But not all rules are created equally. Some were more challenging to create than others. For instance, validation of legal forms in any country worldwide was a tough one. We needed to collect all the legal forms for each country – just imagine thousands of abbreviations and alternative abbreviations. And to make matters worse, data maintainers use totally crazy variants sometimes.
Legal forms, company names, various formats, abbreviated, not abbreviated, partially abbreviated, at front of the name, at the end of the name, somewhere in the middle. We had to come up with a rule that could validate all these formats, which was no easy feat.
Despite the challenges, I am most proud of the worldwide coverage of tax and business identifiers in our rule set. These rules cover not only the format of the identifiers but also calculate check digits and check their existence against business registers. These rules are essential because they ensure high-quality data when it enters the system.
Benefits of using data quality rules
If you're a lonely wolf without data quality rules, you're in for a tough ride. You'll spend years in workshops, discussing budgets and priorities, and iterating with IT departments. But with our ready-to-use ruleset and dashboard, you don't have to go through all that. Our ruleset ensures high-quality data when data enters the system, and it's configurable and extendable by your own rules.
The quantitative benefits of using our rules are substantial.
If each rule specification, research, and implementation, including testing and user acceptance tests, costs around one week of effort, you're saving 2000x5 days = 10,000 days = 45 FTE for one year. These are costs nobody directly sees, but they are implicit in the roles.
The qualitative benefits of using our rules are also significant. Our rules ensure quality, transparency, and avoid crazy discussions and lost time.
You don't have to go through all the workshops, endless discussions, and iterations. Not only does it save time and effort, but it also ensures the quality and transparency of data, so you can focus on your core business. And with CDQ's ready-to-use ruleset and configurable dashboard, the implementation process is made much simpler and more efficient.
Data quality rules are essential for any organization that aims to achieve high-quality data. As I reflect on my journey with CDQ, I'm proud of the work we've done in creating and improving our ruleset, and I'm excited to see how we can continue to innovate and enhance our offering in the years to come.
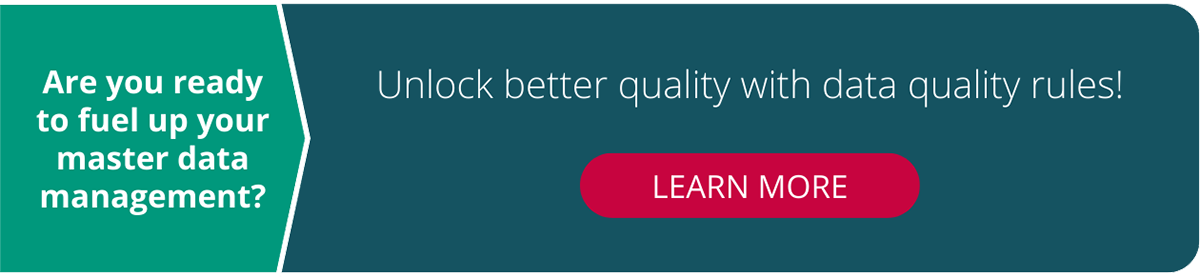
Get our e-mail!
Related blogs
Master Data Management meets AI: work smarter, not harder
Let's be honest, master data management (MDM) often feels like a Sisyphean task. Wrangling inconsistent data, battling data decay, and striving for a single…
How AI and MDM work together to drive business success
In today's fast-paced world, Artificial Intelligence (AI) is becoming a must-have for making smart decisions, automating tasks, and discovering hidden insights.…
Trust, automation, and the future of master data management
Master data management (MDM) is a vital, albeit often underappreciated, foundation of modern business operations. When done right, it ensures smooth processes,…